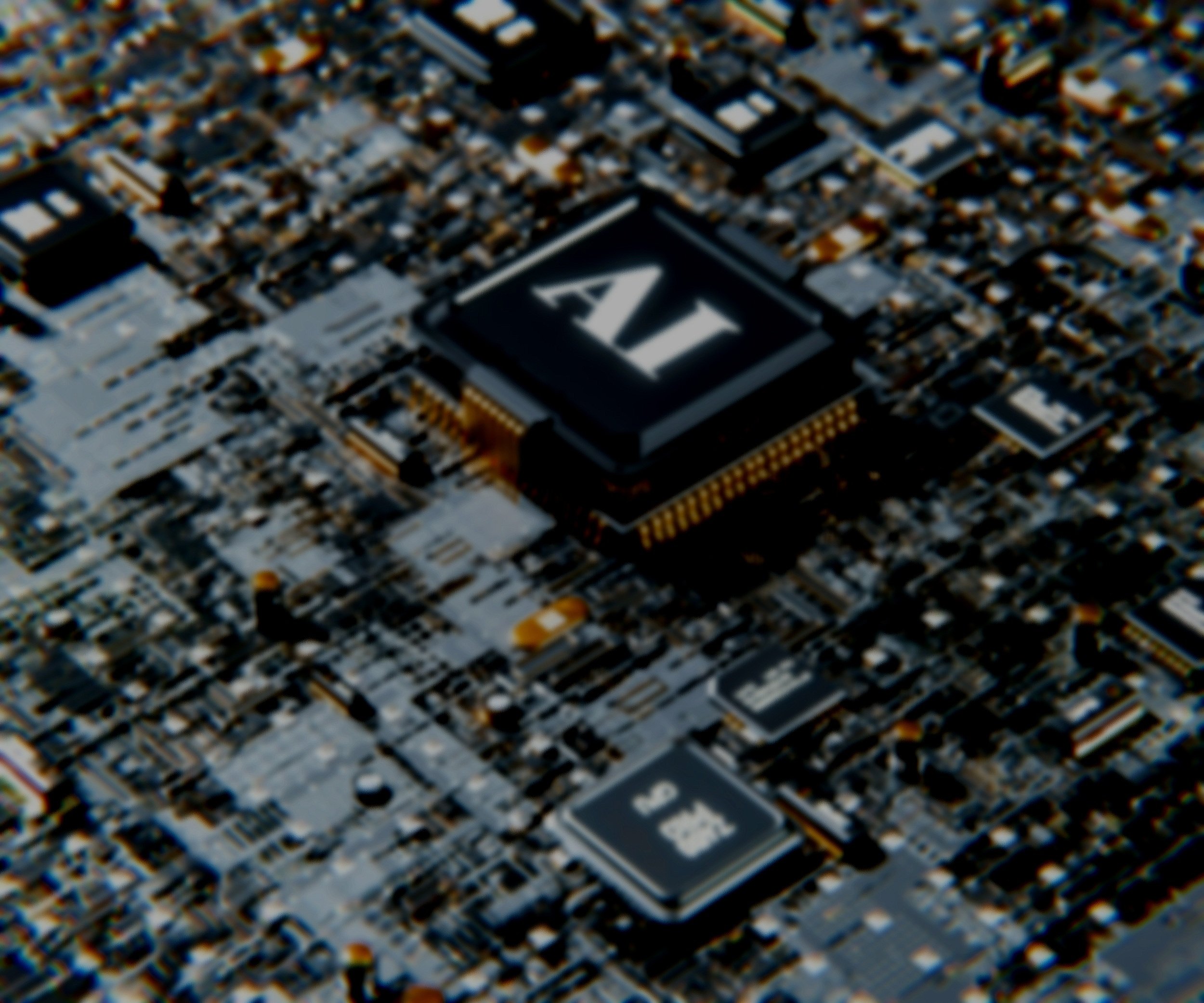
Generative Artificial Intelligence
Imagine an AI system that can create differential diagnoses, simulate complex clinical scenarios, or even generate synthetic data for drug trials. Generative AI, a subset of artificial intelligence, is making this vision a reality. From creating realistic medical imaging to streamlining clinical workflows, generative AI is poised to redefine the healthcare landscape. But how does it work, and what challenges must we navigate to harness its full potential?
What is Generative AI?
Generative AI refers to algorithms capable of creating new multimodal content—be it text, images, audio, or even videos—by learning from existing data. In healthcare, its applications are both vast and profound. Unlike traditional AI models designed for classification or prediction, generative AI can produce outputs that mimic the complexity of human decision-making. This makes it an invaluable tool for addressing medicine’s most pressing challenges, such as reducing diagnostic errors, personalizing therapies, and accelerating research.
Machine Learning (ML), Deep Learning (DL), Generstive AI (GenAI)…Where does ChatGPT fit in?
-
AI is the broad field of creating machines or systems that can replicate human intelligence enabling them to perform tasks such as reasoning, learning, and problem-solving.
The history of artificial intelligence dates back to the mid-20th century, with foundational concepts emerging from the work of pioneering figures such as Alan Turing and John McCarthy. In 1956, the term "artificial intelligence" was coined during a conference at Dartmouth College, marking the beginning of AI as a formal field of study.
-
A subset of artificial intelligence that enables systems to learn from data and improve their performance over time without explicit programming.
By analyzing vast amounts of information, machine learning algorithms can identify patterns, make predictions, and enhance decision-making processes across various fields. Applications range from healthcare, where it aids in disease diagnosis and treatment personalization, to finance, where it helps in fraud detection and risk management.
-
A subset of machine learning that uses artificial neural networks to analyze and interpret complex data.
By mimicking the human brain's interconnected neuron structure, deep learning algorithms can process vast amounts of information, identifying patterns and making predictions with remarkable accuracy.
-
Generative AI leverages deep learning techniques to create new content, such as images, text, and audio, that closely resembles existing data.
By using advanced neural networks, generative AI can analyze vast datasets and learn intricate patterns, enabling it to produce outputs that are both innovative and realistic.
-
A type of GenAI designed to understand, generate, and manipulate human language.
Their ability to generate coherent and contextually relevant responses has implications across various fields, including education, healthcare, and customer service.
-
Developed by OpenAI, GPT is capable of understanding and generating human-like text based on the context and input it receives.
Generative: Able to create new content
Pre-Trained: Learned from terabytes of data
Transformer: Able to understand this unstructured data due to the novel Transformer architecture.
What’s so special about the Transformers Architecture?
The transformer architecture revolutionized AI by enabling models to process and generate human-like text with a deep understanding of context, predicting coherent sequences of words from a user's input.
Earlier neural networks processed words one at a time in sequence. If you only think about that word and what came immediately before and after it. You might miss how everything fits together.
Transformers analyze entire inputs simultaneously, allowing them to grasp relationships between all words at once, much like how humans understand sentences as a whole.
This ability opened the door for AI to learn from large unstructured datasets. Using extensive datasets from the internet, books, articles, and other sources, GPT learns the statistical relationships between words, phrases, and sentences. When given a prompt, it predicts the next word in a sequence by calculating the probabilities of possible continuations, selecting those with the highest likelihood to create coherent and contextually relevant text.
Limitations of GPT
While GPT is a powerful tool capable of generating human-like text and providing insights on a wide range of topics, it has limitations that users should be aware of:
Lack of True Understanding: GPT does not "understand" information in the way humans do. It generates responses based on patterns in its training data rather than genuine comprehension or reasoning.
Accuracy and Reliability: The model's responses are limited by the quality of its training data, which may include outdated, incomplete, or inaccurate information. GPT does not inherently recognize whether the content it generates contains falsehoods, misrepresentations, or inaccuracies, nor can it verify its truthfulness. As a probabilistic algorithm, it may produce varying responses to the same prompt, which could include repeated errors, improvements to prior inaccuracies, or entirely new mistakes. This variability poses challenges for reliability and reproducibility, underscoring the need for continuous human oversight and independent verification of critical information.
Bias and Ethical Concerns: GPT can inadvertently reproduce biases present in its training data, including cultural, gender, or racial biases. Care should be taken to critically evaluate its outputs.
Ethical and Legal Risks: Using GPT in healthcare raises concerns about patient privacy, informed consent, and accountability for adverse outcomes stemming from its recommendations.